The Secrets of Salient Object Segmentation
Author(s)
Li, Yin; Hou, Xiaodi; Koch, Christof; Rehg, James M.; Yuille, Alan L.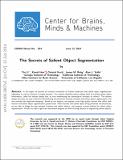
DownloadCBMM-Memo-014.pdf (1.586Mb)
Terms of use
Metadata
Show full item recordAbstract
In this paper we provide an extensive evaluation of fixation prediction and salient object segmentation algorithms as well as statistics of major datasets. Our analysis identifies serious design flaws of existing salient object benchmarks, called the dataset design bias, by over emphasising the stereotypical concepts of saliency. The dataset design bias does not only create the discomforting disconnection between xations and salient object segmentation, but also misleads the algorithm designing. Based on our analysis, we propose a new high quality dataset that offers both fixation and salient object segmentation ground-truth. With fixations and salient object being presented simultaneously, we are able to bridge the gap between fixations and salient objects, and propose a novel method for salient object segmentation. Finally, we report significant benchmark progress on three existing datasets of segmenting salient objects.
Date issued
2014-06-13Publisher
Center for Brains, Minds and Machines (CBMM), arXiv
Citation
arXiv:1406.2807v2
Series/Report no.
CBMM Memo Series;014
Keywords
Fixation Prediction, Machine Learning, Object Recognition
Collections
The following license files are associated with this item: