Human-Machine CRFs for Identifying Bottlenecks in Holistic Scene Understanding
Author(s)
Mottaghi, Roozbeh; Fidler, Sanja; Yuille, Alan L.; Urtasun, Raquel; Parikh, Devi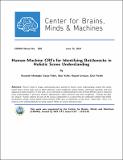
DownloadCBMM-Memo-020.pdf (1.885Mb)
Terms of use
Metadata
Show full item recordAbstract
Recent trends in image understanding have pushed for holistic scene understanding models that jointly reason about various tasks such as object detection, scene recognition, shape analysis, contextual reasoning, and local appearance based classifiers. In this work, we are interested in understanding the roles of these different tasks in improved scene understanding, in particular semantic segmentation, object detection and scene recognition. Towards this goal, we “plug-in” human subjects for each of the various components in a state-of-the-art conditional random field model. Comparisons among various hybrid human-machine CRFs give us indications of how much “head room” there is to improve scene understanding by focusing research efforts on various individual tasks.
Date issued
2014-06-15Publisher
Center for Brains, Minds and Machines (CBMM), arXiv
Citation
arXiv:1406.3906
Series/Report no.
CBMM Memo Series;020
Keywords
Object Recognition, Scene Recognition, Vision
Collections
The following license files are associated with this item: