Deep Captioning with Multimodal Recurrent Neural Networks (m-RNN)
Author(s)
Mao, Junhua; Xu, Wei; Yang, Yi; Wang, Jiang; Huang, Zhiheng; Yuille, Alan L.; ... Show more Show less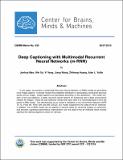
DownloadCBMM-Memo-033.pdf (839.4Kb)
Terms of use
Metadata
Show full item recordAbstract
In this paper, we present a multimodal Recurrent Neural Network (m-RNN) model for generating novel image captions. It directly models the probability distribution of generating a word given previous words and an image. Image captions are generated according to this distribution. The model consists of two sub-networks: a deep recurrent neural network for sentences and a deep convolutional network for images. These two sub-networks interact with each other in a multimodal layer to form the whole m-RNN model. The effectiveness of our model is validated on four benchmark datasets (IAPR TC-12, Flickr 8K, Flickr 30K and MS COCO). Our model outperforms the state-of-the-art methods. In addition, the m-RNN model can be applied to retrieval tasks for retrieving images or sentences, and achieves significant performance improvement over the state-of-the-art methods which directly optimize the ranking objective function for retrieval.
Date issued
2015-05-07Publisher
Center for Brains, Minds and Machines (CBMM), arXiv
Citation
arXiv:1412.6632
Series/Report no.
CBMM Memo Series;033
Keywords
multimodal Recurrent Neural Network (m-RNN), Artificial Intelligence, Computer Language
Collections
The following license files are associated with this item: