An integrative predictive model for hospital acquired complications
Author(s)
Liu, Jenny, M. Eng. Massachusetts Institute of Technology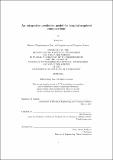
DownloadFull printable version (2.878Mb)
Other Contributors
Massachusetts Institute of Technology. Department of Electrical Engineering and Computer Science.
Advisor
Gil Alterovitz.
Terms of use
Metadata
Show full item recordAbstract
Hospital Acquired Complications (HACs) are a serious problem affecting modern day healthcare institutions. It is estimated that in US hospitals, HACs cause an approximately 10% increase in total inpatient hospital costs. With US hospital spending totaling nearly $900 billion per year, the tremendous damages caused by HACs is no small matter. Early detection and prevention of HACs could greatly reduce strains on the US healthcare system and improve mortality rates. Here we show a machine-learning model for predicting the occurrence of HACs using clinical data limited to short periods following Intensive Care Unit (ICU) admission. In addition, we also identify several keystone features that demonstrate high predictive power HACs during certain time periods following patient admission. Based on our research, we can reduce excessive hospital costs due to HAC by at least $10 billion annually. We can also reduce the number of excessive hospital stay days by 4.6 million days, and potentially reduce patient mortality by at least 10k patients. The classifiers and features analyzed in this study show high promise of being able to be used for accurate prediction of HACs in clinical settings long before the complication symptoms are manifested. These findings could provide a great aid to doctors and other healthcare professionals in containing the damages caused by HACs in healthcare institutions nationwide.
Description
Thesis: M. Eng., Massachusetts Institute of Technology, Department of Electrical Engineering and Computer Science, 2015. This electronic version was submitted by the student author. The certified thesis is available in the Institute Archives and Special Collections. Cataloged from student-submitted PDF version of thesis. Includes bibliographical references (pages 45-50).
Date issued
2015Department
Massachusetts Institute of Technology. Department of Electrical Engineering and Computer SciencePublisher
Massachusetts Institute of Technology
Keywords
Electrical Engineering and Computer Science.