Towards an epidemiology of gentrification : modeling urban change as a probabilistic process using k-means clustering and Markov models
Author(s)
Royall, Emily Binet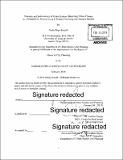
DownloadFull printable version (14.89Mb)
Alternative title
Modeling urban change as a probabilistic process using k-means clustering and Markov models
Other Contributors
Massachusetts Institute of Technology. Department of Urban Studies and Planning.
Advisor
Andrea Chegut.
Terms of use
Metadata
Show full item recordAbstract
Gentrification is viewed as both as a tool and a force--as a systematized vehicle for classbased oppression and racism, and an empirical force of change based on social, environmental and economic interactions. This complexity makes it challenging for researchers to study the impact of gentrification, for planners to anticipate the effects of gentrification with planning policy, and for developers to foresee investment outcomes. Current planning policy addresses the symptoms of gentrification, without defining the underlying construct of the process. This thesis examines latent constructs of gentrification through a data-driven process that identifies emergent states of change and assigns them to a Markov process, i.e. a process that assigns probabilities to potential "state" changes over time. For census block groups in four boroughs of New York City, this model takes three steps: 1) cluster census block groups into latent states defined by ACS socioeconomic and demographic data, 2) derive a Markov model by tracking transitions between states over time, and 3) validate the model by testing predictions against historic data and qualitative documentation. Using this process I was able to find emergent typologies of urban change, locate gentrifying neighborhoods without any spatial input, and uncover sequences of patterns that reliably predict socioeconomic outcomes at the census block group level. Through the design of a machine learning framework for gentrification I reflect on the importance of using algorithms that learn rather than reproduce assumptions, value of distilling large and complex data relationships into nuanced intuitions, and challenges of embedding computational modeling in political frameworks.
Description
Thesis: M.C.P., Massachusetts Institute of Technology, Department of Urban Studies and Planning, 2016. Vita. Cataloged from PDF version of thesis. Includes bibliographical references.
Date issued
2016Department
Massachusetts Institute of Technology. Department of Urban Studies and PlanningPublisher
Massachusetts Institute of Technology
Keywords
Urban Studies and Planning.