Revealing disease-associated pathways and components by systematic integration of large-scale biological data
Author(s)
Pirhaji, Leila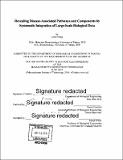
DownloadFull printable version (13.31Mb)
Other Contributors
Massachusetts Institute of Technology. Department of Biological Engineering.
Advisor
Ernest Fraenkel.
Terms of use
Metadata
Show full item recordAbstract
While technological advances have enabled measurements of thousands of molecules simultaneously, the data from each technology can only show a single-view of biological processes. Capturing a complete picture of these processes requires integrating data of different types, including clinical data, genomics, transcriptomics, proteomics and metabolomics. Here, we have demonstrated novel computational approaches for integrating a variety of biological data and used these methods to study Huntington's disease (HD). First, we established a computational approach for combining transcriptomics with qualitative, ordinal clinical information. Such data are available for a variety of diseases, but are rarely used in conjunction with molecular data. We adapted an ordinal regression model to analyze gene expression data from HD brains in conjunction with their grade of neuronal loss. This approach identified the SGPLl-encoded enzyme (SPL) as a potential therapeutic target for HD. Continuing our data-driven approach, we discovered the dysregulation of pathways associated with SPL and inferred molecular mechanisms by which SPL inhibition exerts protective effects. Then, we demonstrated a novel network-based, machine-learning algorithm for integrative analysis of untargeted metabolomic data. Metabolites are small molecules whose levels directly show cellular phenotypes. Despite their potential, the integrative analysis of metabolomic data has been limited because of challenges in metabolite identification. To address these challenges, we have developed a pioneering method for interpreting the large-scale metabolomic data in the context of other molecules such as proteins. We used our method to infer novel dysregulated pathways in a model of HD and experimentally verified our predictions. These two methods are extremely general and can be applied to a variety of diseases. As the costs of generating high-throughput data decrease, we anticipate that our approaches will have growing relevance to the discovery of therapeutic strategies for precision medicine.
Description
Thesis: Ph. D., Massachusetts Institute of Technology, Department of Biological Engineering, 2016. Cataloged from PDF version of thesis. Includes bibliographical references (pages 129-141).
Date issued
2016Department
Massachusetts Institute of Technology. Department of Biological EngineeringPublisher
Massachusetts Institute of Technology
Keywords
Biological Engineering.