Bayesian inference of chemical reaction networks
Author(s)
Galagali, Nikhil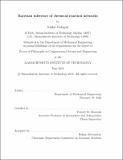
DownloadFull printable version (10.68Mb)
Other Contributors
Massachusetts Institute of Technology. Department of Mechanical Engineering.
Advisor
Youssef M. Marzouk.
Terms of use
Metadata
Show full item recordAbstract
The development of chemical reaction models aids system design and optimization, along with fundamental understanding, in areas including combustion, catalysis, electrochemistry, and biology. A systematic approach to building reaction network models uses available data not only to estimate unknown parameters, but to also learn the model structure. Bayesian inference provides a natural approach for this data-driven construction of models. Traditional Bayesian model inference methodology is based on evaluating a multidimensional integral for each model. This approach is often infeasible for reaction network inference, as the number of plausible models can be very large. An alternative approach based on model-space sampling can enable large-scale network inference, but its efficient implementation presents many challenges. In this thesis, we present new computational methods that make large-scale nonlinear network inference tractable. Firstly, we exploit the network-based interactions of species to design improved "between-model" proposals for Markov chain Monte Carlo (MCMC). We then introduce a sensitivity-based determination of move types which, when combined with the network-aware proposals, yields further sampling efficiency. These algorithms are tested on example problems with up to 1000 plausible models. We find that our new algorithms yield significant gains in sampling performance, with almost two orders of magnitude reduction in the variance of posterior estimates. We also show that by casting network inference as a fixed-dimensional problem with point-mass priors, we can adapt existing adaptive MCMC methods for network inference. We apply this novel framework to the inference of reaction models for catalytic reforming of methane from a set of ~/~ 32000 possible models and real experimental data. We find that the use of adaptive MCMC makes large-scale inference of reaction networks feasible without the often extensive manual tuning that is required with conventional approaches. Finally, we present an approximation-based method that allows sampling over very large model spaces whose exploration remains prohibitively expensive with ex-act sampling methods. We run an MCMC algorithm over model indicators and for each visited model approximate the model evidence via Laplace's method. Limited and sparse available data tend to produce multi-modal posteriors over the model indicators. To perform inference in this setting, we develop a population-based approximate model inference MCMC algorithm. Numerical tests on problems with around 109 models demonstrate the superiority of our population-based algorithm over single-chain MCMC approaches.
Description
Thesis: Ph. D., Massachusetts Institute of Technology, Department of Mechanical Engineering, 2016. Cataloged from PDF version of thesis. Includes bibliographical references (pages 189-198).
Date issued
2016Department
Massachusetts Institute of Technology. Department of Mechanical EngineeringPublisher
Massachusetts Institute of Technology
Keywords
Mechanical Engineering.