Optimizing hybrid vehicles : battery pack design, energy management, and collaborative learning
Author(s)
Erb, Dylan C. (Dylan Charles)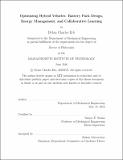
DownloadFull printable version (8.805Mb)
Other Contributors
Massachusetts Institute of Technology. Department of Mechanical Engineering.
Advisor
Sanjay E. Sarma.
Terms of use
Metadata
Show full item recordAbstract
Hybrid vehicles are designed to operate efficiently using data from within the bounds of that single vehicle. With limited access to outside information, hybrid control systems can react to external stimuli but cannot prepare ahead of time, which limits the overall efficiency. This thesis looks beyond the scope of traditional automotive systems to increase efficiency and boost performance of hybrid vehicles while decreasing cost and maximizing drivability. I cover three main subject areas which are all focused on hybrid vehicle optimization: battery pack design for low cost thermal management, energy management of blended battery packs for weight minimization, and collaborative learning for improved energy management. The outputs of this thesis include insights into the minimization of air-cooling costs in battery packs, a better understanding of the blended battery pack design space, and in-vehicle applications of collaborative learning. First, I have advanced the understanding of how cell-level properties affect the overall costs of air-cooling in battery packs and have shown how to minimize those costs. Through both simulation and analytical studies, I illustrated that cell size and geometry can be selected to balance the tradeoff between average and delta temperature constraints. At the intersection of these two constraints, a cell size can be found that results in the minimum cost of air-cooling for the parameters of that application. While the simulation results take significant time to compute, the proposed analytical method can be used to narrow down a design space in a fraction of the time through direct calculation. Second, I have shown how blended battery packs can be sized for a given application and have identified what applications will benefit most from tribridization. Using integer programming for a lower bound, a dynamic programming based method for minimum feasibility, and a peak shaving heuristic as a baseline, I carried out a large study. In this experiment, I quantified how much room for improvement there is for a variety of hybrid applications through the pack-level combination of different energy storage technologies. Based on the results, I extracted rules of thumb for understanding when blended battery packs can improve over uniform packs. Finally, I have demonstrated how collaborative learning can benefit energy management for hybrid vehicles. I illustrated how the drivability and fuel efficiency of current micro hybrids suffer from the automatic starting and stopping of the engine for relatively short idles. By defining a traffic model and comparing that to realworld datasets, I identified parameters that are most useful in predicting idle time of vehicles in traffic. Using these predictors in combination with machine learning classifiers, I quantified the potential for a Smart/Stop system that harnesses collaborative learning to improve over current automatic engine start/stop performance. The result is a system that can significantly increase drivability while maintaining or even improving upon baseline fuel economy.
Description
Thesis: Ph. D., Massachusetts Institute of Technology, Department of Mechanical Engineering, 2016. This electronic version was submitted by the student author. The certified thesis is available in the Institute Archives and Special Collections. Cataloged from student-submitted PDF version of thesis. Includes bibliographical references (pages 197-207).
Date issued
2016Department
Massachusetts Institute of Technology. Department of Mechanical EngineeringPublisher
Massachusetts Institute of Technology
Keywords
Mechanical Engineering.