Reduced-space Gaussian process regression forecast for nonlinear dynamical systems
Author(s)
Wan, Zhong Yi, Ph. D. Massachusetts Institute of Technology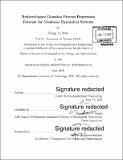
DownloadFull printable version (10.23Mb)
Other Contributors
Massachusetts Institute of Technology. Computation for Design and Optimization Program.
Advisor
Themistoklis P. Sapsis.
Terms of use
Metadata
Show full item recordAbstract
In this thesis work, we formulate a reduced-order data-driven strategy for the efficient probabilistic forecast of complex high-dimensional dynamical systems for which data-streams are available. The first step of this method consists of the reconstruction of the vector field in a reduced-order subspace of interest using Gaussian Process Regression (GPR). GPR simultaneously allows for the reconstruction of the vector field, as well as the estimation of the local uncertainty. The latter is due to i) the local interpolation error and ii) due to the truncation of the high-dimensional phase space and it analytically quantified in terms of the GPR hyperparameters. The second step involves the formulation of stochastic models that explicitly take into account the reconstructed dynamics and their uncertainty. For regions of the attractor where the training data points are not sufficiently dense for GPR to be effective an adaptive blended scheme is formulated that guarantees correct statistical steady state properties. We examine the effectiveness of the proposed method to complex systems including the Lorenz 63, Lorenz 96, the Kuramoto-Sivashinsky, as well as a prototype climate model. We also study the performance of the proposed approach as the intrinsic dimensionality of the system attractor increases in highly turbulent regimes.
Description
Thesis: S.M., Massachusetts Institute of Technology, Computation for Design and Optimization Program, 2016. Cataloged from PDF version of thesis. Includes bibliographical references (pages 93-97).
Date issued
2016Department
Massachusetts Institute of Technology. Computation for Design and Optimization ProgramPublisher
Massachusetts Institute of Technology
Keywords
Computation for Design and Optimization Program.