Where do hypotheses come from?
Author(s)
Dasgupta, Ishita; Schulz, Eric; Gershman, Samuel J.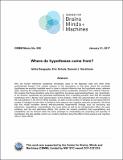
DownloadCBMM-Memo-056-v2.pdf (733.3Kb)
Terms of use
Metadata
Show full item recordAbstract
Why are human inferences sometimes remarkably close to the Bayesian ideal and other times systematically biased? One notable instance of this discrepancy is that tasks where the candidate hypotheses are explicitly available result in close to rational inference over the hypothesis space, whereas tasks requiring the self-generation of hypotheses produce systematic deviations from rational inference. We propose that these deviations arise from algorithmic processes approximating Bayes' rule. Specifically in our account, hypotheses are generated stochastically from a sampling process, such that the sampled hypotheses form a Monte Carlo approximation of the posterior. While this approximation will converge to the true posterior in the limit of infinite samples, we take a small number of samples as we expect that the number of samples humans take is limited by time pressure and cognitive resource constraints. We show that this model recreates several well-documented experimental findings such as anchoring and adjustment, subadditivity, superadditivity, the crowd within as well as the self-generation effect, the weak evidence, and the dud alternative effects. Additionally, we confirm the model's prediction that superadditivity and subadditivity can be induced within the same paradigm by manipulating the unpacking and typicality of hypotheses, in 2 experiments.
Date issued
2016-10-24Publisher
Center for Brains, Minds and Machines (CBMM)
Series/Report no.
CBMM Memo Series;056
Keywords
Bayes' Rule, Monte Carlo approximation, superadditivity, subadditivity