Information-sharing models for computational genetics
Author(s)
Edwards, Matthew Douglas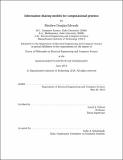
DownloadFull printable version (7.350Mb)
Other Contributors
Massachusetts Institute of Technology. Department of Electrical Engineering and Computer Science.
Advisor
David K. Gifford.
Terms of use
Metadata
Show full item recordAbstract
Modern genetics has been transformed by a dramatic explosion of data. As sample sizes and the number of measured data types grow, the need for computational methods tailored to deal with these noisy and complex datasets increases. In this thesis, we develop and apply integrated computational and biological approaches for two genetic problems. First, we build a statistical model for genetic mapping using pooled sequencing, a powerful and efficient technique for rapidly unraveling the genetic basis of complex traits. Our approach explicitly models the pooling process and genetic parameters underlying the noisy observed data, and we use it to calculate accurate intervals that contain the targeted regions of interest. We show that our model outperforms simpler alternatives that do not use all available marker data in a principled way. We apply this model to study several phenotypes in yeast, including the genetic basis of the surprising phenomenon of strain-specific essential genes. We demonstrate the complex genetic basis of many of these strain-specific viability phenotypes and uncover the influence of an inherited virus in modifying their effects. Second, we design a statistical model that uses additional functional information describing large sets of genetic variants in order to predict which variants are likely to cause phenotypic changes. Our technique is able to learn complicated relationships between candidate features and can accommodate the additional noise introduced by training on groups of candidate variants, instead of single labeled variants. We apply this model to a large genetic mapping study in yeast by collecting multiple genome-wide functional measurements. By using our model, we demonstrate the importance of several molecular phenotypes in predicting genetic impact. The common themes in this thesis are the development of computational models that accurately reflect the underlying biological processes and the integration of carefully controlled biological experiments to test and utilize our new models.
Description
Thesis: Ph. D., Massachusetts Institute of Technology, Department of Electrical Engineering and Computer Science, 2016. This electronic version was submitted by the student author. The certified thesis is available in the Institute Archives and Special Collections. Cataloged from student-submitted PDF version of thesis. Includes bibliographical references (pages 97-105).
Date issued
2016Department
Massachusetts Institute of Technology. Department of Electrical Engineering and Computer SciencePublisher
Massachusetts Institute of Technology
Keywords
Electrical Engineering and Computer Science.