A uniform representation for visual concepts
Author(s)
Rakover, Nicolas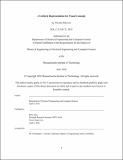
DownloadFull printable version (5.973Mb)
Other Contributors
Massachusetts Institute of Technology. Department of Electrical Engineering and Computer Science.
Advisor
Boris Katz.
Terms of use
Metadata
Show full item recordAbstract
We present a method for learning visually-grounded word meanings, given as input a set of videos paired with natural-language sentences describing them. Our method uses a uniform feature representation for all words and word types rather than relying on handcrafted features specific to each word. We learn words in a weakly-supervised manner, with no need for annotated bounding boxes around objects of interest. We encode words as Hidden Markov models such that word models can be composed according to a sentence's semantic structure to efficiently recognize events in videos. We use a discriminative variant of Baum-Welch to learn the parameters for our word models, and demonstrate that our approach is able to learn words capturing appearance, spatial relations, and temporal dynamics.
Description
Thesis: M. Eng., Massachusetts Institute of Technology, Department of Electrical Engineering and Computer Science, 2016. This electronic version was submitted by the student author. The certified thesis is available in the Institute Archives and Special Collections. Cataloged from student-submitted PDF version of thesis. Includes bibliographical references (pages 54-55).
Date issued
2016Department
Massachusetts Institute of Technology. Department of Electrical Engineering and Computer SciencePublisher
Massachusetts Institute of Technology
Keywords
Electrical Engineering and Computer Science.