Using behavioral analytics and machine learning to improve churn management
Author(s)
Aberg Cobo, Ignacio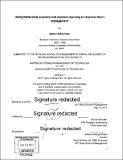
DownloadFull printable version (7.423Mb)
Other Contributors
Technology and Policy Program.
Advisor
Duncan Simester.
Terms of use
Metadata
Show full item recordAbstract
New trends are shaping the telecommunications, media and technology (TMT) industries. Consumers are demanding to be connected anytime to hundreds of thousands of applications that are one click away. In addition, loyalty levels are decreasing and customers do not hesitate to switch providers if they do not receive value for their money. Because of this, churn management is a key driver of profits. However, few companies excel at churn management and most underestimate its impact. The thesis is focused on describing a technological solution targeted to improve churn management capabilities within companies that belong to the TMT sector and explore the opportunities and hurdles of selling this kind of solution in a B2B context. The hypothesis is that a world class churn management solution can effectively deploy statistical models to score customers by their likelihood to churn and execute targeted treatments for each segment through the operator service channels. The study will focus on how behavioral analytics and machine learning can increase customer's life time value and boost margins in TMT companies. Throughout the research, I will describe the best practices within the industry to establish a state of the art churn management solution.
Description
Thesis: S.M., Massachusetts Institute of Technology, Sloan School of Management, 2017. Cataloged from PDF version of thesis. Includes bibliographical references (page 36).
Date issued
2017Department
Sloan School of ManagementPublisher
Massachusetts Institute of Technology
Keywords
Sloan School of Management., Technology and Policy Program.