Convergence of regulatory mutations into oncogenic pathways across multiple tumor types
Author(s)
Murugadoss, Karthik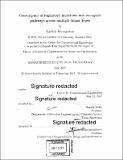
DownloadFull printable version (5.436Mb)
Other Contributors
Massachusetts Institute of Technology. Computation for Design and Optimization Program.
Advisor
Manolis Kellis.
Terms of use
Metadata
Show full item recordAbstract
Cancer sequencing efforts have largely focused on profiling somatic variants in the protein-coding genome and characterizing their functional impact. In this study, we develop a computational pipeline to identify non-coding mutational drivers across multiple tumor types. We describe the non-coding mutational profiles of 854 samples, spread across 15 tumor types, in the context of their respective tissue type-specific reference epigenomes, using recent pan-cancer whole-genome sequencing data. We develop a novel method to detect significantly recurrent non-coding mutations by reestimating the background mutation density while adjusting for epigenomic covariates. Existing databases on enhancer-gene links allow us to capture the convergence of disparate mutations onto downstream target genes. We then systematically identify key immunomodulatory and tumor-suppressive genes enriched for non-coding mutations in their regulatory neighborhood and evaluate these in a pan-cancer context. Taken together, we show that low-frequency alterations converge into high-frequency recurrent events on downstream targets through tissue-specific regulatory interactions.
Description
Thesis: S.M., Massachusetts Institute of Technology, Computation for Design and Optimization Program, 2017. Cataloged from PDF version of thesis. Includes bibliographical references (pages 65-74).
Date issued
2017Department
Massachusetts Institute of Technology. Computation for Design and Optimization ProgramPublisher
Massachusetts Institute of Technology
Keywords
Computation for Design and Optimization Program.