Towards more biologically plausible deep learning and visual processing
Author(s)
Liao, Qianli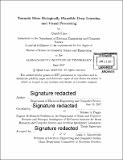
DownloadFull printable version (10.33Mb)
Other Contributors
Massachusetts Institute of Technology. Department of Electrical Engineering and Computer Science.
Advisor
Tomaso A. Poggio.
Terms of use
Metadata
Show full item recordAbstract
Over the last decade, we have witnessed tremendous successes of Artificial Neural Networks (ANNs) on solving a wide range of Al tasks. However, there is considerably less development in understanding the biological neural networks in primate cortex. In this thesis, I try to bridge the gap between artificial and biological neural networks. I argue that it would be beneficial to build ANNs that are both biologically-plausible and well-performing, since they may serve as models for the brain and guide neuroscience research. On the other hand, developing a biology-compatible framework for ANNs makes it possible to borrow ideas from neuroscience to improve the performance of AI systems. I discuss several aspects of modern ANNs that can be made more consistent with biology: (1) the backpropagation learning algorithm (2) ultra-deep neural networks (e.g., ResNet, He et al., 2016) for visual processing (3) Batch Normalization (Ioffe and Szegedy, 2015). For each of the three aspects, I propose biologically-plausible modifications of the ANN models to make them more implementable by the brain while maintaining (or even improving) their performance.
Description
Thesis: S.M., Massachusetts Institute of Technology, Department of Electrical Engineering and Computer Science, 2017. Cataloged from PDF version of thesis. Includes bibliographical references (pages 85-91).
Date issued
2017Department
Massachusetts Institute of Technology. Department of Electrical Engineering and Computer SciencePublisher
Massachusetts Institute of Technology
Keywords
Electrical Engineering and Computer Science.