Eye tracking for the iPhone using deep learning
Author(s)
Kannan, Harini D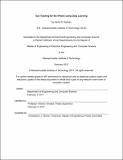
DownloadFull printable version (2.887Mb)
Other Contributors
Massachusetts Institute of Technology. Department of Electrical Engineering and Computer Science.
Advisor
Antonio Torralba.
Terms of use
Metadata
Show full item recordAbstract
Accurate eye trackers on the market today require specialized hardware and are very costly. If eye-tracking could be available for free to anyone with a camera phone, the potential impact could be great. For example, free eye tracking assistive technology could help people with paralysis to regain control of their day-to-day activities, such as sending email. The first part of this thesis describes the software implementation and the current performance metrics of the original iTracker neural network, which was published in the CVPR 2016 paper "Eye Tracking for Everyone." This original iTracker network had a 1.86 centimeter error for eye tracking on the iPhone. The second part of this thesis describes the efforts towards creating an improved neural network with a smaller centimeter error. A new error of 1.66 centimeters (11% improvement from the previous benchmark) was achieved using ensemble learning with the ResNet10 model with batch normalization.
Description
Thesis: M. Eng., Massachusetts Institute of Technology, Department of Electrical Engineering and Computer Science, 2017. This electronic version was submitted by the student author. The certified thesis is available in the Institute Archives and Special Collections. Cataloged from student-submitted PDF version of thesis. Includes bibliographical references (page 45).
Date issued
2017Department
Massachusetts Institute of Technology. Department of Electrical Engineering and Computer SciencePublisher
Massachusetts Institute of Technology
Keywords
Electrical Engineering and Computer Science.