Long short-term memory recurrent neural networks for classification of acute hypotensive episodes
Author(s)
Jaffe, Alexander Scott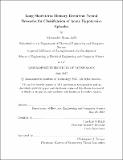
DownloadFull printable version (537.8Kb)
Alternative title
LSTM RNN for classification of AHE
Other Contributors
Massachusetts Institute of Technology. Department of Electrical Engineering and Computer Science.
Advisor
Una-May O'Reilly.
Terms of use
Metadata
Show full item recordAbstract
An acute hypotensive episode (AHE) is a life-threatening condition durich which a patient's mean arterial blood pressure drops below 60 mmHG for a period of 30 minutes. This thesis presents the development and evaluation of a series of Long short-term memory recurrent neural network (LSTM RNN) models which predict whether a patient will experience an AHE or not based on a time series of mean arterial blood pressure (ABP). A 2-layer, 128-hidden unit LSTM RNN trained with rmsprop and dropout regularization achieves sensitivity of 78% and specificity of 98%.
Description
Thesis: M. Eng., Massachusetts Institute of Technology, Department of Electrical Engineering and Computer Science, 2017. This electronic version was submitted by the student author. The certified thesis is available in the Institute Archives and Special Collections. Cataloged from student-submitted PDF version of thesis. Includes bibliographical references (pages 37-39).
Date issued
2017Department
Massachusetts Institute of Technology. Department of Electrical Engineering and Computer SciencePublisher
Massachusetts Institute of Technology
Keywords
Electrical Engineering and Computer Science.