Parallel and distributed MCMC inference using Julia
Author(s)
Yu, Angel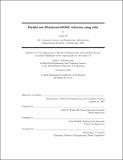
DownloadFull printable version (2.828Mb)
Alternative title
Parallel and distributed Markov chain Monte Carlo inference using Julia
Other Contributors
Massachusetts Institute of Technology. Department of Electrical Engineering and Computer Science.
Advisor
John W. Fisher III and Oren Freifeld.
Terms of use
Metadata
Show full item recordAbstract
Machine learning algorithms are often computationally intensive and operate on large datasets. Being able to eciently learn models on large datasets holds the future of machine learning. As the speed of serial computation stalls, it is necessary to utilize the power of parallel computing in order to better scale with the growing complexity of algorithms and the growing size of datasets. In this thesis, we explore the use of Julia, a fairly new high level programming language that lends itself to easy parallelization over multiple CPU cores as well as multiple machines, on Markov chain Monte Carlo (MCMC) inference algorithms. First, we take existing algorithms and implement them in Julia. We focus on MCMC inference using Continuous Piecewise-Affine Based (CPAB) transformations and a parallel MCMC sampler for Dirichlet Process Mixture Models (DPMM). Instead of parallelizing over multiple cores on a single machine, our Julia implementations extend existing implementations by parallelizing over multiple machines. We compare our implementation with these existing implementations written in more traditional programming languages. Next, we develop a model Projections Dirichlet Process Gaussian Mixture Model (PDP-GMM) which relaxes the assumption that the draws from a Dirichlet Process Gaussian Mixture Model (DP-GMM) are directly observed. We extend our DPMM Julia implementation and present a few applications of this model.
Description
Thesis: M. Eng., Massachusetts Institute of Technology, Department of Electrical Engineering and Computer Science, 2016. This electronic version was submitted by the student author. The certified thesis is available in the Institute Archives and Special Collections. Cataloged from student-submitted PDF version of thesis. Includes bibliographical references (pages 71-72).
Date issued
2016Department
Massachusetts Institute of Technology. Department of Electrical Engineering and Computer SciencePublisher
Massachusetts Institute of Technology
Keywords
Electrical Engineering and Computer Science.