Learning diseases from data : a disease space odyssey
Author(s)
Haslam, Bryan (Bryan Todd)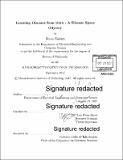
DownloadFull printable version (24.28Mb)
Alternative title
Disease space odyssey
Other Contributors
Massachusetts Institute of Technology. Department of Electrical Engineering and Computer Science.
Advisor
Luis Perez-Breva.
Terms of use
Metadata
Show full item recordAbstract
Recent commitments to enhance the use of data for learning in medicine provide the opportunity to apply instruments and abstractions from computational learning theory to systematize learning in medicine. The hope is to accelerate the rate at which we incorporate knowledge and improve healthcare quality. In this thesis, we work to bring further clarity to the ways in which computational learning theory can be applied to update the collective knowledge about diseases. Researchers continually study and learn about the complex nature of the human body. They summarize this knowledge with the best possible set of diseases and how those diseases relate to each other. We draw on computational learning theory to understand and broaden this form of collective learning. This mode of collective learning is regarded as unsupervised learning, as no disease labels are initially available. In unsupervised learning, variance is typically reduced to find an optimal function to organize the data. A significant challenge that remains is how to measure variance in the definition of diseases in a comprehensive way. Variance in the definition of a disease introduces a systematic error in both basic and clinical research. If measured, it would also be possible to use computers to efficiently minimize variance, providing a great opportunity for learning by utilizing medical data. In this thesis, we demonstrate that it is possible to estimate variance in the disease taxonomy, effectively estimating an error bar for the current definitions of diseases. We do so using the history of the disease taxonomy and comparing it with a variety of external data sets that relate diseases to attributes such as symptoms, drugs and genes. We demonstrate that variance can be significant over relatively short time periods. We further present methods for updating the disease taxonomy by reducing variance based on external disease data sets. This makes it possible to automatically incorporate information contained in disease data sets into the disease taxonomy. The approach also makes it possible to use expert information encoded in the taxonomy to systematically transfer knowledge and update other biomedical data sets that are often sparse (e.g. - symptoms associated with diseases). A natural question stemming from these results is how granular does data need to be to make improvements? For instance, is patient-level data necessary to enable learning at the macro level of disease? Or are there strategies to extract information from other kinds of data to alleviate the need for very granular data. We show that detailed, patient-level data is not necessarily needed to extract detailed biological data. We do so by comparing disease relationships learned from clinical trial metadata to disease relationships learned from a detailed genetic database and show we can achieve similar results. This result shows that we can use currently available data and take advantage of computational learning to improve disease learning, which suggests a new avenue to improving patient outcomes. By reducing variance within diseases using data available today, we can quickly update the space of diseases to be more precise. Precise diseases lead to better learning in other areas of medicine and ultimately improved healthcare quality.
Description
Thesis: Ph. D., Massachusetts Institute of Technology, Department of Electrical Engineering and Computer Science, 2017. Cataloged from PDF version of thesis. Includes bibliographical references (pages 253-280).
Date issued
2017Department
Massachusetts Institute of Technology. Department of Electrical Engineering and Computer SciencePublisher
Massachusetts Institute of Technology
Keywords
Electrical Engineering and Computer Science.