Flexible models for understanding and optimizing complex populations
Author(s)
Mueller, Jonas Weylin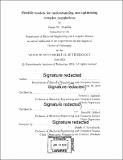
DownloadFull printable version (16.60Mb)
Other Contributors
Massachusetts Institute of Technology. Department of Electrical Engineering and Computer Science.
Advisor
Tommi S. Jaakkola and David K. Gifford.
Terms of use
Metadata
Show full item recordAbstract
Data analysis is often driven by the goals of understanding or optimizing some population of interest. The first of these two objectives aims to produce insights regarding characteristics of the underlying population, often to facilitate scientific understanding. Crucially, this requires models which produce results that are highly interpretable to the analyst. On the other hand, notions of interpretability are not necessarily as central for determining how to optimize populations, where the aim is to build data-driven systems which learn how to act upon individuals in a manner that maximally improves certain outcomes of interest across the population. In this thesis, we develop interpretable yet flexible modeling frameworks for addressing the former goal, as well as black-box nonparametric methods for addressing the latter. Throughout, we demonstrate various empirical applications of our algorithms, primarily in the biological context of modeling gene expression in large cell populations. For better understanding populations, we introduce two nonparametric models that can accurately reflect interesting characteristics of complex distributions without reliance on restrictive assumptions, while simultaneously remaining highly interpretable through their use of the Wasserstein (optimal transport) metric to summarize changes over an entire population. One approach is principal differences analysis, a projection-based technique that interpretably characterizes differences between two arbitrary high-dimensional probability distributions. Another approach is the TRENDS model, which quantifies the underlying effects of temporal progression in an evolving sequence of distributions that also vary due to confounding noise. While the aforementioned techniques fall under the frequentist regime, we subsequently present a Bayesian framework for the task of optimizing populations. Drawing upon the Gaussian process toolkit, our method learns how to best conservatively intervene upon heterogeneous populations in settings with limited data and substantial uncertainty about the underlying relationship between actions and outcomes.
Description
Thesis: Ph. D., Massachusetts Institute of Technology, Department of Electrical Engineering and Computer Science, 2018. Cataloged from PDF version of thesis. Includes bibliographical references (pages 143-152).
Date issued
2018Department
Massachusetts Institute of Technology. Department of Electrical Engineering and Computer SciencePublisher
Massachusetts Institute of Technology
Keywords
Electrical Engineering and Computer Science.