Single units in a deep neural network functionally correspond with neurons in the brain: preliminary results
Author(s)
Arend, Luke; Han, Yena; Schrimpf, Martin; Bashivan, Pouya; Kar, Kohitij; Poggio, Tomaso; DiCarlo, James J.; Boix, Xavier; ... Show more Show less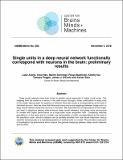
DownloadCBMM-Memo-093.pdf (2.991Mb)
Metadata
Show full item recordAbstract
Deep neural networks have been shown to predict neural responses in higher visual cortex. The mapping from the model to a neuron in the brain occurs through a linear combination of many units in the model, leaving open the question of whether there also exists a correspondence at the level of individual neurons. Here we show that there exist many one-to-one mappings between single units in a deep neural network model and neurons in the brain. We show that this correspondence at the single- unit level is ubiquitous among state-of-the-art deep neural networks, and grows more pronounced for models with higher performance on a large-scale visual recognition task. Comparing matched populations—in the brain and in a model—we demonstrate a further correspondence at the level of the population code: stimulus category can be partially decoded from real neural responses using a classifier trained purely on a matched population of artificial units in a model. This provides a new point of investigation for phenomena which require fine-grained mappings between deep neural networks and the brain.
Date issued
2018-11-02Publisher
Center for Brains, Minds and Machines (CBMM)
Series/Report no.
CBMM Memo Series;093