Towards building active defense for software applications
Author(s)
Perumal, Zara (Zara Alexandra)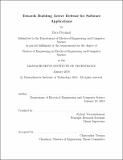
DownloadFull printable version (4.965Mb)
Other Contributors
Massachusetts Institute of Technology. Department of Electrical Engineering and Computer Science.
Advisor
Kalyan Veeramchaneni.
Terms of use
Metadata
Show full item recordAbstract
Over the last few years, cyber attacks have become increasingly sophisticated. In an effort to defend themselves, corporations often look to machine learning, aiming to use the large amount of data collected on cyber attacks and software systems to defend systems at scale. Within the field of machine learning in cybersecurity, PDF malware is a popular target of study, as the difficulty of classifying malicious files makes it a continuously eective method of attack. The obstacles are many: Datasets change over time as attackers change their behavior, and the deployment of a malware detection system in a resource-constrained environment has minimum throughput requirements, meaning that an accurate but time-consuming classier cannot be deployed. Recent work has also shown how automated malicious file creation methods are being used to evade classication. Motivated by these challenges, we propose an active defender system to adapt to evasive PDF malware in a resource-constrained environment. We observe this system to improve the f₁ score from 0.17535 to 0.4562 over five stages of receiving PDF files that the system considers unlabeled. Furthermore, average classication time per le is low across all 5 stages, and is reduced from an average of 1.16908 seconds per le to 1.09649 seconds per le. Beyond classifying malware, we provide a general active defender framework that can be used to deploy decision systems for a variety of resource-constrained adversarial problems.
Description
Thesis: M. Eng., Massachusetts Institute of Technology, Department of Electrical Engineering and Computer Science, 2018. This electronic version was submitted by the student author. The certified thesis is available in the Institute Archives and Special Collections. Cataloged from student-submitted PDF version of thesis. Includes bibliographical references (pages 85-88).
Date issued
2018Department
Massachusetts Institute of Technology. Department of Electrical Engineering and Computer SciencePublisher
Massachusetts Institute of Technology
Keywords
Electrical Engineering and Computer Science.