Robotic object pose estimation with deep neural networks
Author(s)
Wu, Jimmy, M. Eng. Massachusetts Institute of Technology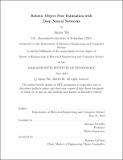
DownloadFull printable version (2.729Mb)
Other Contributors
Massachusetts Institute of Technology. Department of Electrical Engineering and Computer Science.
Advisor
Antonio Torralba.
Terms of use
Metadata
Show full item recordAbstract
In this work, we introduce pose interpreter networks for 6-DoF object pose estimation. In contrast to other CNN-based approaches to pose estimation that require expensively-annotated object pose data, our pose interpreter network is trained entirely on synthetic data. We use object masks as an intermediate representation to bridge real and synthetic. We show that when combined with a segmentation model trained on RGB images, our synthetically-trained pose interpreter network is able to generalize to real data. Our end-to-end system for object pose estimation runs in real-time (20 Hz) on live RGB data, without using depth information or ICP refinement.
Description
Thesis: M. Eng., Massachusetts Institute of Technology, Department of Electrical Engineering and Computer Science, 2018. This electronic version was submitted by the student author. The certified thesis is available in the Institute Archives and Special Collections. Cataloged from student-submitted PDF version of thesis. Includes bibliographical references (pages 39-45).
Date issued
2018Department
Massachusetts Institute of Technology. Department of Electrical Engineering and Computer SciencePublisher
Massachusetts Institute of Technology
Keywords
Electrical Engineering and Computer Science.