Information provision in rating systems and traffic systems
Author(s)
Makhdoumi, Ali (Makhdoumi Kakhaki)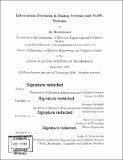
DownloadFull printable version (8.741Mb)
Other Contributors
Massachusetts Institute of Technology. Department of Electrical Engineering and Computer Science.
Advisor
Asuman Ozdaglar and Daron Acemoglu.
Terms of use
Metadata
Show full item recordAbstract
This thesis studies information provision in two contexts: online rating systems and traffic information systems. In the first part of the thesis, we develop a model of Bayesian learning from online reviews and investigate the conditions for asymptotic learning of the quality of a product. Moreover, we characterize the speed of learning under different rating systems and characterize the impact of information provision on the speed of learning. In the model, a sequence of potential customers after observing the ratings of the product, and based on their ex ante valuation, decide whether to purchase. If they purchase, the true quality of the product, their ex ante valuation and ex post valuation determine their overall satisfaction. Given the rating system of the platform, they decide to leave a review as a function of their overall satisfaction. We study learning dynamics under two classes of rating systems: full history, where customers see the full history of reviews, and summary statistics, where the platform reports some summary statistics of past reviews. In both cases, we characterize the asymptotic speed of learning and show that the incentivizes of the platform is aligned with maximizing the speed of learning. We then study the design of rating systems in terms of information collection and information provision schemes. In particular, we identify situations in which providing more information leads to slower learning. In the second part of the thesis, we develop a framework for systematically analyzing how changes in the information sets of users in a traffic network (e.g., due to route guidance systems) impact the traffic equilibrium, and show the conditions under which even those with access to additional information may suffer greater congestion. To this regard, we first introduce a new class of congestion games in which users have differing information sets about the available edges and can only use routes consisting of edges in their information set. We then define the notion of information constrained Wardrop equilibrium for this class of congestion games and establish its existence and uniqueness. Finally, we turn to our main question formulated in the forms of Informational Braess' Paradox (IBP), which extends the classic Braess' Paradox in traffic equilibria. IBP asks whether users receiving additional information can become worse off. We provide a comprehensive answer to this question by providing a tight characterization of network topologies under which IBP emerges.
Description
Thesis: Ph. D., Massachusetts Institute of Technology, Department of Electrical Engineering and Computer Science, 2018. Cataloged from PDF version of thesis. Includes bibliographical references (pages 133-141).
Date issued
2018Department
Massachusetts Institute of Technology. Department of Electrical Engineering and Computer SciencePublisher
Massachusetts Institute of Technology
Keywords
Electrical Engineering and Computer Science.