Evaluating parameter optimization in locality-sensitive hashing for high-dimensional physiological waveforms
Author(s)
Chakradhar, Vineel A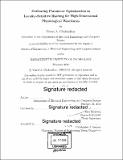
DownloadFull printable version (6.608Mb)
Other Contributors
Massachusetts Institute of Technology. Department of Electrical Engineering and Computer Science.
Advisor
Erik Hemberg.
Terms of use
Metadata
Show full item recordAbstract
We develop and evaluate a theoretical architecture to inform parameter choice for locality-sensitive hashing methods used towards identifying similarity in physiological waveform time-series data. The goal is to achieve increased probability of successful patient outcomes in emergency rooms by tackling the problem of efficient information retrieval within massive, high-dimensional medical datasets. To solve this problem, we explore the relationship between a number of data inputs and elements of locality-sensitive hashing schemes in order to drive optimal choice of parameters throughout the pipeline from raw data to locality-sensitive hashing output. We achieve significant increases in retrieval times while generally maintaining the prediction accuracy achieved by naive retrieval methodologies.
Description
Thesis: M. Eng., Massachusetts Institute of Technology, Department of Electrical Engineering and Computer Science, 2018. Cataloged from PDF version of thesis. "The pagination listed in the Table of Contents does not correlate with actual page numbering"--Disclaimer Notice page. Includes bibliographical references (pages 71-72).
Date issued
2018Department
Massachusetts Institute of Technology. Department of Electrical Engineering and Computer SciencePublisher
Massachusetts Institute of Technology
Keywords
Electrical Engineering and Computer Science.