Net-PPI : mapping the human interactome with machine learned models
Author(s)
Schreiber, Kfir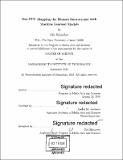
DownloadFull printable version (4.917Mb)
Alternative title
Mapping the human interactome with machine learned models
Net-protein-protein interactions : mapping the human interactome with machine learned models
Other Contributors
Program in Media Arts and Sciences (Massachusetts Institute of Technology)
Advisor
Joseph M. Jacobson.
Terms of use
Metadata
Show full item recordAbstract
The miracle of life is only possible thanks to a wide range of biochemical interactions between assortments of molecular agents. Amidst these agents, which enable all cellular activities, proteins are undoubtedly among the most important groups. Proteins facilitate countless intra- and inter-cellular functions, from regulation of gene expression to immune responses to muscle contraction, but they rarely act in isolation. These are the interactions between proteins, known as protein-protein interactions or PPIs, which sustain the fundamental role of proteins in all living organisms. PPIs are also central to the study of diseases and development of therapeutics. Aberrant human PPIs are the primary cause of many life-threatening conditions, such as Alzheimer, Creutzfeldt-Jakob, and cancer; making the regulation of PPI activities a promising direction for pharmaceutical development. Despite the indisputable importance of PPIs, so far only a tiny fraction of all human PPIs has been discovered, and our current understanding of the core mechanisms and primary functionalities is insufficient. While computational methods in general and machine learning in particular showed encouraging potential to address this challenge, their application in real-life has been limited. To mitigate this gap and make sure computational results perform as well in real-life, we introduce a set of gold-standard machine learning practices called NetPPI. The contributions of this thesis include NetPPI, a minimally-biased, carefully curated dataset of experimentally detected PPIs for training and evaluation of machine learning models; a comprehensive study of protein sequence representations for use with discriminative models; and data splitting methodology for machine learning purposes. We also present the Bilinear PPI model for state-of-the-art PPI prediction. Finally, we propose fundamental biological insight on the nature of PPIs, based on performance analysis of different prediction models.
Description
Thesis: S.M., Massachusetts Institute of Technology, School of Architecture and Planning, Program in Media Arts and Sciences, 2018. Cataloged from PDF version of thesis. Includes bibliographical references (pages 61-69).
Date issued
2018Department
Program in Media Arts and Sciences (Massachusetts Institute of Technology)Publisher
Massachusetts Institute of Technology
Keywords
Program in Media Arts and Sciences ()