Applicability of deep learning approaches to non-convex optimization for trajectory-based policy search
Author(s)
Verkuil, Robert(Robert H.)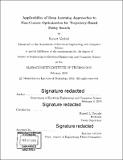
Download1102057655-MIT.pdf (7.892Mb)
Other Contributors
Massachusetts Institute of Technology. Department of Electrical Engineering and Computer Science.
Advisor
Russell L. Tedrake.
Terms of use
Metadata
Show full item recordAbstract
Trajectory optimization is a powerful tool for determining good control sequences for actuating dynamical systems. In the past decade, trajectory optimization has been successfully used to train and guide policy search within deep neural networks via optimizing over many trajectories simultaneously, subject to a shared neural network policy constraint. This thesis seeks to understand how this specific formulation converges in comparison to known globally optimal policies for simple classical control systems. To do so, results from three lines of experimentation are presented. First, trajectory optimization control solutions are compared against globally optimal policies determined via value iteration on simple control tasks. Second, three systems built for parallelized, non-convex optimization across trajectories with a shared neural network constraint are described and analyzed. Finally, techniques from deep learning known to improve convergence speed and quality in non-convex optimization are studied when applied to both the shared neural networks and the trajectories used to train them.
Description
Thesis: M. Eng., Massachusetts Institute of Technology, Department of Electrical Engineering and Computer Science, 2019 Cataloged from PDF version of thesis. Includes bibliographical references (pages 75-76).
Date issued
2019Department
Massachusetts Institute of Technology. Department of Electrical Engineering and Computer SciencePublisher
Massachusetts Institute of Technology
Keywords
Electrical Engineering and Computer Science.