Adaptive robust model predictive control for nonlinear systems
Author(s)
Lopez, Brett Thomas.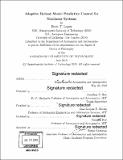
Download1119667757-MIT.pdf (10.90Mb)
Other Contributors
Massachusetts Institute of Technology. Department of Aeronautics and Astronautics.
Advisor
Jonathan P. How.
Terms of use
Metadata
Show full item recordAbstract
Modeling error and external disturbances can severely degrade the performance of Model Predictive Control (MPC) in real-world scenarios. Robust MPC (RMPC) addresses this limitation by optimizing over control policies but at the expense of computational complexity. An alternative strategy, known as tube MPC, uses a robust controller (designed offline) to keep the system in an invariant tube centered around a desired nominal trajectory (generated online). While tube MPC regains tractability, there are several theoretical and practical problems that must be solved for it to be used in real-world scenarios. First, the decoupled trajectory and control design is inherently suboptimal, especially for systems with changing objectives or operating conditions. Second, no existing tube MPC framework is able to capture state-dependent uncertainty due to the complexity of calculating invariant tubes, resulting in overly-conservative approximations. And third, the inability to reduce state-dependent uncertainty through online parameter adaptation/estimation leads to systematic error in the trajectory design. This thesis aims to address these limitations by developing a computationally tractable nonlinear tube MPC framework that is applicable to a broad class of nonlinear systems.
Description
Thesis: Ph. D., Massachusetts Institute of Technology, Department of Aeronautics and Astronautics, 2019 Cataloged from PDF version of thesis. Includes bibliographical references (pages 115-124).
Date issued
2019Department
Massachusetts Institute of Technology. Department of Aeronautics and AstronauticsPublisher
Massachusetts Institute of Technology
Keywords
Aeronautics and Astronautics.