Big data analysis interrogating raw material variability and the impact on process performance
Author(s)
Lopez Marino, Maria Emilia.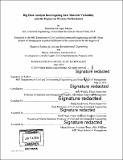
Download1119722244-MIT.pdf (13.11Mb)
Other Contributors
Sloan School of Management.
Massachusetts Institute of Technology. Department of Civil and Environmental Engineering.
Leaders for Global Operations Program.
Advisor
Roy Welsch and Philip Gschwend.
Terms of use
Metadata
Show full item recordAbstract
Within the biopharmaceutical industry, material sciences is a rapidly growing field to continue to ensure reliable production and delivery of medicines. Consequently, there is an on-going need to evaluate and assess new materials, driven by novel process technologies and new modalities. Finding a solution to technically assess the impact of raw material attributes on the manufacturing process represents a significant opportunity to ensure supply. This study seeks to develop a novel predictive framework to assess the impact of raw material variability on the performance of commercial biologic manufacturing processes. Through machine learning techniques, the impact of two strategic raw materials is evaluated by modeling and predicting the outcomes of critical process performance variables and product quality attributes. As part of this research, we aimed to equip Amgen Inc. with a novel learning tool delivering the potential to uncover a deeper level of material variability understanding which: (1) ensures reliable supply through consistent performance, (2) provides insights to material attributes, and (3) delivers the capability to solve material-related investigations more efficiently. Models trained via machine learning showed 89 % average accuracy on predictions for new data. In addition to the demonstrated predictive power, the models developed were highly interpretable and illustrated correlations with several material attributes. Henceforth, the framework developed is the starting point of a novel methodology towards input material variability understanding. The predictive framework was implemented as a web-tool and is currently being piloted at Amgen Inc. The modular design of the predictive models and the web-tool enable the application to other production processes and associated raw materials, and could be generalized across the industry.
Description
Thesis: M.B.A., Massachusetts Institute of Technology, Sloan School of Management, in conjunction with the Leaders for Global Operations Program at MIT, 2019 Thesis: S.M., Massachusetts Institute of Technology, Department of Civil and Environmental Engineering, in conjunction with the Leaders for Global Operations Program at MIT, 2019 Cataloged from PDF version of thesis. Includes bibliographical references (pages 96-102).
Date issued
20192019
Department
Sloan School of Management; Massachusetts Institute of Technology. Department of Civil and Environmental Engineering; Leaders for Global Operations ProgramPublisher
Massachusetts Institute of Technology
Keywords
Sloan School of Management., Civil and Environmental Engineering., Leaders for Global Operations Program.