Generalizable neural network representations of patient state in the intensive care unit
Author(s)
Gong, Maryann M.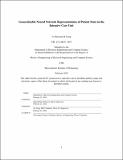
Download1126543154-MIT.pdf (461.0Kb)
Other Contributors
Massachusetts Institute of Technology. Department of Electrical Engineering and Computer Science.
Advisor
John Guttag and Jen Gong.
Terms of use
Metadata
Show full item recordAbstract
Understanding changes in physiology in patients in the Intensive Care Unit (ICU) is important in determining care decisions. Machine learning algorithms have been used to model patient physiology to predict patient outcomes and administration of interventions. These predictions can be made directly on the raw patient data extracted from electronic health records. However, this data can be high dimensional with extraneous information. Neural networks, and in particular, autoencoders and sequence-to-sequence models, can be used to extract the important attributes of this time-series data without manual feature selection. In this work, we explore how learned encoded representations of physiological time-series and events time-series can be used to effectively predict outcomes on a variety of tasks. We compare the representations extracted from sequence-to-sequence models with representations extracted from autoencoders. We evaluate these representations on the task of predicting patient mortality and first onset of ventilator and vasopressor interventions. Our best representations achieve AUCs of 0.83, 0.91, and 0.91 on the tasks of mortality prediction, vasopressor first onset prediction, and ventilator first onset prediction, which is comparable to the performances using the raw features.
Description
This electronic version was submitted by the student author. The certified thesis is available in the Institute Archives and Special Collections. Thesis: M. Eng., Massachusetts Institute of Technology, Department of Electrical Engineering and Computer Science, 2018 Cataloged from student-submitted PDF version of thesis. "February 2018." Includes bibliographical references (pages 28-29).
Date issued
2018Department
Massachusetts Institute of Technology. Department of Electrical Engineering and Computer SciencePublisher
Massachusetts Institute of Technology
Keywords
Electrical Engineering and Computer Science.