Bootstrapping fully-automatic temporal fetal brain segmentation in volumetric MRI time series
Author(s)
Zhang, Lawrence,M. Eng.Massachusetts Institute of Technology.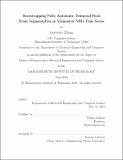
Download1127291738-MIT.pdf (1.221Mb)
Other Contributors
Massachusetts Institute of Technology. Department of Electrical Engineering and Computer Science.
Advisor
Polina Golland.
Terms of use
Metadata
Show full item recordAbstract
We present a method for bootstrapping training data for the task of segmenting fetal brains in volumetric MRI time series data. Temporal analysis of MRI images requires accurate segmentation across frames, despite large amounts of unpredictable motion. We use the predicted segmentations of a baseline model and leverage anatomical structure of the fetal brain to automatically select the "good frames" that have accurate segmentations. We use these good frames to bootstrap further model training. We also introduce a novel temporal segmentation model that predicts segmentations using a history of previous segmentations, thus utilizing the temporal nature of the data. Our results show that these two approaches do not provide conclusive improvements to the quality of segmentations. Further exploration into the automatic choice of good frames is needed before
Description
This electronic version was submitted by the student author. The certified thesis is available in the Institute Archives and Special Collections. Thesis: M. Eng., Massachusetts Institute of Technology, Department of Electrical Engineering and Computer Science, 2019 Cataloged from student-submitted PDF version of thesis. Includes bibliographical references (pages 41-42).
Date issued
2019Department
Massachusetts Institute of Technology. Department of Electrical Engineering and Computer SciencePublisher
Massachusetts Institute of Technology
Keywords
Electrical Engineering and Computer Science.