Modeling correlations in clinical trial outcomes using machine learning
Author(s)
Chavez-Gehrig, Arturo.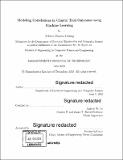
Download1127579518-MIT.pdf (6.274Mb)
Other Contributors
Massachusetts Institute of Technology. Department of Electrical Engineering and Computer Science.
Advisor
Andrew W. Lo.
Terms of use
Metadata
Show full item recordAbstract
This thesis explores the problem of characterizing the covariance of clinical trial outcomes using drug and trial features. The binary nature of FDA approvals makes drug development risky, but approaches in finance theory could better manage that risk, allowing more high potential drugs to be developed. To apply these methods confidently, it is necessary to understand the covariance between projects. The paper outlines several approaches for this task and their theoretical foundations, such as finding the nearest valid covariance matrix, online sequence prediction, and a new approach using function approximation via random forest. This function approximation approach to estimating covariance is implemented and tested on historical clinical trial data.
Description
Thesis: M. Eng., Massachusetts Institute of Technology, Department of Electrical Engineering and Computer Science, 2019 Cataloged from PDF version of thesis. Includes bibliographical references (pages 69-71).
Date issued
2019Department
Massachusetts Institute of Technology. Department of Electrical Engineering and Computer SciencePublisher
Massachusetts Institute of Technology
Keywords
Electrical Engineering and Computer Science.