Multi-modal image registration with unsupervised deep learning
Author(s)
Guo, Courtney K.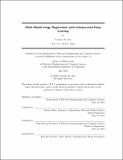
Download1128823285-MIT.pdf (8.778Mb)
Other Contributors
Massachusetts Institute of Technology. Department of Electrical Engineering and Computer Science.
Advisor
Adrian Dalca and John Guttag.
Terms of use
Metadata
Show full item recordAbstract
In this thesis, we tackle learning-based multi-modal image registration. Multi-modal registration, in which two images of dierent modalities need to be aligned to each other, is a difficult yet essential task for medical imaging analysis. Classical methods have been developed for single-modal and multi-modal registration, but are slow because they solve an optimization problem for each pair of images. Recently, deep learning methods for registration have been proposed, and have been shown to shorten registration time by learning a global function to perform registration, which can then be applied quickly on a pair of test images. These methods perform well for single-modal registration but have not yet been extended to the harder task of multi-modal registration. We bridge this gap by implementing classical multi-modal metrics in a differentiable and efficient manner to enable deep image registration for multi-modal data. We nd that our method for multi-modal registration performs significantly better than baselines, in terms of both accuracy and runtime.
Description
This electronic version was submitted by the student author. The certified thesis is available in the Institute Archives and Special Collections. Thesis: M. Eng., Massachusetts Institute of Technology, Department of Electrical Engineering and Computer Science, 2019 Cataloged from student-submitted PDF version of thesis. Includes bibliographical references (pages 37-40).
Date issued
2019Department
Massachusetts Institute of Technology. Department of Electrical Engineering and Computer SciencePublisher
Massachusetts Institute of Technology
Keywords
Electrical Engineering and Computer Science.