Predicting compulsive alcohol drinking
Author(s)
Lee, Jennifer J.,M. Eng.Massachusetts Institute of Technology.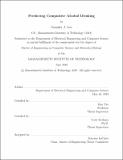
Download1129384832-MIT.pdf (1.553Mb)
Other Contributors
Massachusetts Institute of Technology. Department of Electrical Engineering and Computer Science.
Advisor
Kay Tye and Cody Siciliano.
Terms of use
Metadata
Show full item recordAbstract
Alcohol addiction is a prevalent and complex disorder, with neural underpinnings that warrant further investigation. We devised a novel Pavlovian conditioning task whereby compulsive alcohol drinking behaviors are induced in a mouse model. This model identified individual variations in alcohol intake and compulsive drinking between animals and across time, and showed that medial prefrontal cortex-dorsal periaqueductal gray (mPFC-dPAG) projectors are involved in the encoding of alcohol related stimuli. To elucidate the role of mPFC-dPAG neurons in compulsive alcohol drinking, we first created a transparent signalprocessing method to reliably and accurately detect transients in neuronal traces. This pipeline limits data modification as much as possible, and employs a simple moving average filter and sliding z-score threshold to identify likely events. We also built a transcriptional profile of the mPFC-dPAG neurons via translating ribosome affinity purification (TRAP) followed by RNA sequencing technology, and validated our findings via fluorescent in situ hybridization. Finally, we turned to the online BXD database to build prediction models capable of classifying mice as "low drinkers" or "high drinkers" based on consensus genotypes. Together, these approaches reveal the behavioral and neural characteristics that facilitate the development of alcohol-induced compulsive behaviors.
Description
This electronic version was submitted by the student author. The certified thesis is available in the Institute Archives and Special Collections. Thesis: M. Eng. in Computer Science and Molecular Biology, Massachusetts Institute of Technology, Department of Electrical Engineering and Computer Science, 2019 Cataloged from student-submitted PDF version of thesis. Includes bibliographical references (pages 33-34).
Date issued
2019Department
Massachusetts Institute of Technology. Department of Electrical Engineering and Computer SciencePublisher
Massachusetts Institute of Technology
Keywords
Electrical Engineering and Computer Science.