Scalable large scale visual recognition using multi-label image classification
Author(s)
Huang, Aaron R.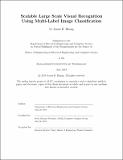
Download1129456299-MIT.pdf (1.179Mb)
Other Contributors
Massachusetts Institute of Technology. Department of Electrical Engineering and Computer Science.
Advisor
Fredo Durand.
Terms of use
Metadata
Show full item recordAbstract
Today, the amount of image categories and labels for visual recognition is growing at an astonishing rate, and it is becoming increasingly impractical to keep up a high level of accuracy across all categories in addition to retraining these deep networks to classify all these new labels along with previous ones. However, we note that the majority of new labels that are being added now are simply subsets and combinations of existing labels, just an extra step of specificity. In this study, we will be looking at creating a model that can be trained on traditional datasets, either single-class or multi-class, but then can be quickly adapted and trained on new multi-class image datasets, in which the class components are part of the original training set, without losing accuracy on the original dataset and not having to train on it either.
Description
This electronic version was submitted by the student author. The certified thesis is available in the Institute Archives and Special Collections. Thesis: M. Eng., Massachusetts Institute of Technology, Department of Electrical Engineering and Computer Science, 2019 Cataloged from student-submitted PDF version of thesis. Includes bibliographical references (pages 50-51).
Date issued
2019Department
Massachusetts Institute of Technology. Department of Electrical Engineering and Computer SciencePublisher
Massachusetts Institute of Technology
Keywords
Electrical Engineering and Computer Science.