Computational characterization of radiation-induced defect dynamics and material response
Author(s)
Jin, Miaomiao.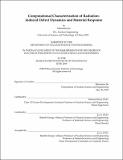
Download1134988592-MIT.pdf (20.49Mb)
Other Contributors
Massachusetts Institute of Technology. Department of Nuclear Science and Engineering.
Advisor
Michael Short.
Terms of use
Metadata
Show full item recordAbstract
Material degradation due to radiation damage poses serious concern on the reliability and durability of any reactor design. To understand material performance under the extreme environments combining high temperature and intense irradiation, the response of radiation damage must be meticulously analyzed, both experimentally and computationally. These efforts will not only bridge the knowledge gap in the fundamental understanding of physical processes, but also allow for prediction of material behavior under a variety of conditions and development of novel materials with superior radiation tolerance. This thesis investigates multiple aspects of radiation damage in materials using various computational methods over a wide range of time and length scale, including atomistic description of defect dynamics, multiscale simulations of radiation processes, and artificial intelligence prediction of material responses based on experimental studies. Firstly, to resolve the fundamental mechanisms of radiation-induced behavior, the traditional molecular dynamics simulations on single-atom damage cascade is extended by developing an algorithm to appropriately introduce numerous consecutive cascades; hence, an experimental dose level on the order of dpa (displacement per atom) can be achieved to enable realistic understanding of observed material responses. It has been utilized to examine the radiation behaviors in solid-solution alloys and nanocrystalline metals such as defect dynamics and grain boundary migration. Secondly, to break the intrinsic limitation of scale in atomistic simulations, a multiscale microstructural evolution framework that links binary-collision approximation, molecular dynamics and cluster dynamics is built to describe mesoscale experimental observations. It is used to successfully explain the non-power-law defect distribution in irradiated tungsten. This tool can be generalized to study the spatial dependent defect evolution in materials under ion irradiation. Finally, to bypass the physics-based complexity of describing materials evolution in real applications, a holistic view enabled by machine learning techniques is utilized, and applied to predict the onset of void swelling in metals with a manually collection of data from experimental studies. The model has generated satisfying results for prediction of unseen data based on material properties and experimental parameters.
Description
This electronic version was submitted by the student author. The certified thesis is available in the Institute Archives and Special Collections. Thesis: Ph. D., Massachusetts Institute of Technology, Department of Nuclear Science and Engineering, 2019 Cataloged from student-submitted PDF version of thesis. Includes bibliographical references (pages 177-200).
Date issued
2019Department
Massachusetts Institute of Technology. Department of Nuclear Science and EngineeringPublisher
Massachusetts Institute of Technology
Keywords
Nuclear Science and Engineering.