Distributionally robust optimization with marginals : theory and applications
Author(s)
Chen, Louis Lester.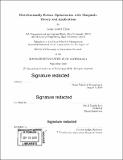
Download1138875683-MIT.pdf (9.913Mb)
Other Contributors
Massachusetts Institute of Technology. Operations Research Center.
Advisor
David Simchi-Levi.
Terms of use
Metadata
Show full item recordAbstract
In this thesis, we consider distributionally robust optimization (DRO) problems in which the ambiguity sets are designed from marginal distribution information - more specifically, when the ambiguity set includes any distribution whose marginals are consistent with given prescribed distributions that have been estimated from data. In the first chapter, we study the class of linear and discrete optimization problems in which the objective coefficients are chosen randomly from a distribution, and the goal is to evaluate robust bounds on the expected optimal value as well as the marginal distribution of the optimal solution. The set of joint distributions is assumed to be specified up to only the marginal distributions. We generalize the primal-dual formulations for this problem from the set of joint distributions with absolutely continuous marginal distributions to arbitrary marginal distributions using techniques from optimal transport theory. While the robust bound is shown to be NP-hard to compute for linear optimization problems, we identify multiple sufficient conditions for polynomial time solvability - one using extended formulations, another exploiting the interaction of combinatorial structure and optimal transport. This generalizes the known tractability results under marginal information from 0-1 polytopes to a class of integral polytopes and has implications on the solvability of distributionally robust optimization problems in areas such as scheduling, which we discuss. In the second chapter, we extend the primal-dual analysis of the previous chapter to the problem of distributionally robust network design. In this problem, the decision maker is to decide on the prepositioning of resources on arcs in a given s-t flow network in anticipation of an adversarys selection of a probability distribution for the arc capacities, aimed to minimize the expected max flow. Again, the adversarys selection is limited to those distributions that are couplings of given are capacity distributions, one for each arc. We show that we can efficiently solve the distributionally robust network design problem in the case of finite-supported marginals. Further, we take advantage of the network setting to efficiently solve for the distribution the adversary responds with. The primal-dual formulation of our previous work takes on a striking form in this study. As one might expect, the form relates to the well-known Max Flow, Min-Cut theorem. And this leads to the intriguing interpretation as a 2-player, zero-sum game wherein player 1 chooses what to set the arc capacities to and player 2 chooses an s-t cut. Essential to our analysis is the finding that the problem of finding the worst-case coupling of the stochastic arc capacities amounts to finding a distribution over the set of s-t cuts- this distribution being among the mixed strategies that player 2 would play in a Nash equilibrium. Furthermore, the support of such a distribution is a nested collection of s-t cuts, which implies an efficiently sized solution. Finally, the third chapter involves work inspired by the daily operations of HEMA supermarket, which is a recently established new retail model by Alibaba Group, China. In a HEMA supermarket store, a single SKU may be presented with demand in the form of multiple channels. The challenge facing HEMA is the question of how many units to stock in total between the warehouse and the store-front in advance of uncertain demand that arises in several consecutive time frames, each 30 minutes long. In this work, we provide the first distributionally robust optimization study in the setting of omnichannel inventory management, wherein we are to make a stocking decision robust to an adversarys choice of coupling of available (marginal) demand distributions by channel and by time frame. The adversarys coupling decision amounts to designing a random mathematical program with equilibrium constraints (MPEC). And we provide both a structural analysis of the adversarys choice of coupling as well as an efficient procedure to find this coupling. In general, the overall distributionally robust stocking problem is non-concave. We provide sufficient conditions on the cost parameters under which this problem becomes concave, and hence tractable. Finally, we conduct experiments with HEMAs data. In these experiments, we compare and contrast the performance of our distributionally robust solution with the performance of a naive Newsvendor-like solution on various SKUs of varying sales volume and number of channels on a 5-hour time window from 2pm - 7pm on weekends. Numerical experiments show that the distributionally robust solutions generally outperform the stochastic Newsvendor-like solution in SKUs exhibiting low-medium sales volume. Furthermore, and interestingly, in all of our experiments, the distributionally robust inventory decision problems presented by the historical data provided by HEMA are in fact concave.
Description
Thesis: Ph. D., Massachusetts Institute of Technology, Sloan School of Management, Operations Research Center, 2019 Cataloged from PDF version of thesis. Includes bibliographical references (pages 121-125).
Date issued
2019Department
Massachusetts Institute of Technology. Operations Research Center; Sloan School of ManagementPublisher
Massachusetts Institute of Technology
Keywords
Operations Research Center.