Counterfactual policy introspection using structural causal models
Author(s)
Oberst, Michael Karl.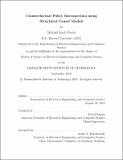
Download1142635604-MIT.pdf (2.526Mb)
Other Contributors
Massachusetts Institute of Technology. Department of Electrical Engineering and Computer Science.
Advisor
David Sontag.
Terms of use
Metadata
Show full item recordAbstract
Inspired by a growing interest in applying reinforcement learning (RL) to healthcare, we introduce a procedure for performing qualitative introspection and `debugging' of models and policies. In particular, we make use of counterfactual trajectories, which describe the implicit belief (of a model) of 'what would have happened' if a policy had been applied. These serve to decompose model-based estimates of reward into specific claims about specific trajectories, a useful tool for 'debugging' of models and policies, especially when side information is available for domain experts to review alongside the counterfactual claims. More specically, we give a general procedure (using structural causal models) to generate counterfactuals based on an existing model of the environment, including common models used in model-based RL. We apply our procedure to a pair of synthetic applications to build intuition, and conclude with an application on real healthcare data, introspecting a policy for sepsis management learned in the recently published work of Komorowski et al. (2018).
Description
This electronic version was submitted by the student author. The certified thesis is available in the Institute Archives and Special Collections. Thesis: S.M., Massachusetts Institute of Technology, Department of Electrical Engineering and Computer Science, 2019 Cataloged from student-submitted PDF version of thesis. Includes bibliographical references (pages 97-102).
Date issued
2019Department
Massachusetts Institute of Technology. Department of Electrical Engineering and Computer SciencePublisher
Massachusetts Institute of Technology
Keywords
Electrical Engineering and Computer Science.