Robust non-Gaussian semantic simultaneous localization and mapping
Author(s)
Doherty, Kevin J.(Automated vehicles software expert)(Kevin Joseph)Massachusetts Institute of Technology.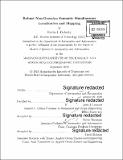
Download1143741104-MIT.pdf (8.924Mb)
Other Contributors
Joint Program in Applied Ocean Science and Engineering.
Massachusetts Institute of Technology. Department of Aeronautics and Astronautics.
Woods Hole Oceanographic Institution.
Advisor
John J. Leonard.
Terms of use
Metadata
Show full item recordAbstract
The recent success of object detection systems motivates object-based representations for robot navigation; i.e. semantic simultaneous localization and mapping (SLAM), in which we aim to jointly estimate the pose of the robot over time as well as the location and semantic class observed objects. A solution to the semantic SLAM problem necessarily addresses the continuous inference problems where am I? and where are the objects?, but also the discrete inference problem what are the objects?. We consider the problem of semantic SLAM under non-Gaussian uncertainty. The most prominent case in which this arises is from data association uncertainty, where we do not know with certainty what objects in the environment caused the measurement made by our sensor. The semantic class of an object can help to inform data association; a detection classified as a door is unlikely to be associated to a chair object. However, detectors are imperfect, and incorrect classification of objects can be detrimental to data association. While previous approaches seek to eliminate such measurements, we instead model the robot and landmark state uncertainty induced by data association in the hopes that new measurements may disambiguate state estimates, and that we may provide representations useful for developing decisionmaking strategies where a robot can take actions to mitigate multimodal uncertainty. The key insight we leverage is that the semantic SLAM problem with unknown data association can be refrained as a non-Gaussian inference problem. We present two solutions to the resulting problem: we first assume Gaussian measurement models, and non-Gaussianity only due to data association uncertainty. We then relax this assumption and provide a method that can cope with arbitrary non-Gaussian measurement models. We show quantitatively on both simulated and real data that both proposed methods have robustness advantages as compared to traditional solutions when data associations are uncertain.
Description
Thesis: S.M., Joint Program in Applied Ocean Physics and Engineering (Massachusetts Institute of Technology, Department of Aeronautics and Astronautics; and the Woods Hole Oceanographic Institution), 2019 Cataloged from PDF version of thesis. Includes bibliographical references (pages 99-103).
Date issued
2019Department
Joint Program in Applied Ocean Science and Engineering; Massachusetts Institute of Technology. Department of Aeronautics and Astronautics; Woods Hole Oceanographic InstitutionPublisher
Massachusetts Institute of Technology
Keywords
Joint Program in Applied Ocean Science and Engineering., Aeronautics and Astronautics., Woods Hole Oceanographic Institution.