Molecular graph Self attention and graph convolution for drug discovery
Author(s)
Bair, Annamarie Elizabeth.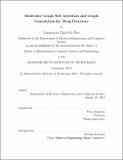
Download1144932843-MIT.pdf (971.3Kb)
Other Contributors
Massachusetts Institute of Technology. Department of Electrical Engineering and Computer Science.
Advisor
Peter Szolovits.
Terms of use
Metadata
Show full item recordAbstract
Drug development is an important, but complicated and expensive process. By utilizing the power of deep learning, we aim to improve the current process of drug development. We model molecules as undirected graphs and use graph convolutions and self-attention to predict molecular properties. With a series of ablation studies, we demonstrate the added value of several key components in our network. We analyze two standard datasets: BBBP, which includes classication data on whether molecules pass the blood-brain barrier, and ClinTox, which includes toxicity information. Using our architecture, we are able to match state of the art performance on the BBBP prediction task.
Description
This electronic version was submitted by the student author. The certified thesis is available in the Institute Archives and Special Collections. Thesis: M. Eng., Massachusetts Institute of Technology, Department of Electrical Engineering and Computer Science, 2019 Cataloged from student-submitted PDF version of thesis. Includes bibliographical references (pages 55-57).
Date issued
2019Department
Massachusetts Institute of Technology. Department of Electrical Engineering and Computer SciencePublisher
Massachusetts Institute of Technology
Keywords
Electrical Engineering and Computer Science.