An entropy-based approach to network attack classification with deep neural network
Author(s)
Do, Emily H.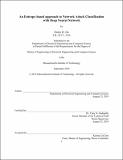
Download1145005007-MIT.pdf (2.633Mb)
Other Contributors
Massachusetts Institute of Technology. Department of Electrical Engineering and Computer Science.
Advisor
Vijay N. Gadepally.
Terms of use
Metadata
Show full item recordAbstract
Detecting and classifying attacks on computer networks is a significant challenge for network providers and users. This thesis project builds a deep neural network to detect and classify network attacks. Our approach based on the hypothesis that each type of network attacks generates a distinguishable change in the entropies of certain features of network flows. To generate a training and validation dataset, synthetic attacks of different types and levels of intensity are injected to the MAWI dataset, which contains captured raw network traffic from an Internet backbone link. Experimental results show that our machine learning model can achieve a high accuracy for network attacks which intensity is as low as 6% of the original traffic. This result is very promising, considering the fact that the amount of traffic in the Internet backbone link is substantial. This work also evaluates and quantifies the model performances on different attack types and the levels of intensity of these attacks.
Description
This electronic version was submitted by the student author. The certified thesis is available in the Institute Archives and Special Collections. Thesis: M. Eng., Massachusetts Institute of Technology, Department of Electrical Engineering and Computer Science, 2019 Cataloged from student-submitted PDF version of thesis. Includes bibliographical references (pages 61-65).
Date issued
2019Department
Massachusetts Institute of Technology. Department of Electrical Engineering and Computer SciencePublisher
Massachusetts Institute of Technology
Keywords
Electrical Engineering and Computer Science.