Causal structure discovery from incomplete data
Author(s)
Squires, Chandler(Chandler B.)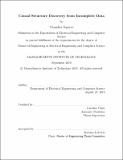
Download1145169413-MIT.pdf (1.360Mb)
Other Contributors
Massachusetts Institute of Technology. Department of Electrical Engineering and Computer Science.
Advisor
Caroline Uhler.
Terms of use
Metadata
Show full item recordAbstract
Causal structure learning is a fundamental tool for building a scientific understanding of the way a system works. However, in many application areas, such as genomics, the information necessary for current causal structure learning algorithms does not match the information that researchers can actually access, for example when the algorithm requires knowledge of intervention targets but the interventions have off-target effects. In this thesis, we developed, implemented, and tested a novel algorithm for discovering a causal DAG from observational and interventional data, when the intervention targets are either partially or completely unknown. We relate the algorithm to the recently introduced Joint Causal Inference framework. Finally, we evaluate the performance of the algorithm on synthetic datasets and demonstrated its ability to outperform current state-of-the-art causal structure learning algorithms which assume known intervention targets.
Description
This electronic version was submitted by the student author. The certified thesis is available in the Institute Archives and Special Collections. Thesis: M. Eng., Massachusetts Institute of Technology, Department of Electrical Engineering and Computer Science, 2019 Cataloged from student-submitted PDF version of thesis. Includes bibliographical references (pages 43-44).
Date issued
2019Department
Massachusetts Institute of Technology. Department of Electrical Engineering and Computer SciencePublisher
Massachusetts Institute of Technology
Keywords
Electrical Engineering and Computer Science.