Disentangling time constant and time dependent hidden state in time series with variational Bayesian inference
Author(s)
Grullon, Dylan Emanuel Centeno.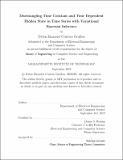
Download1149026032-MIT.pdf (15.85Mb)
Other Contributors
Massachusetts Institute of Technology. Department of Electrical Engineering and Computer Science.
Advisor
Duane S. Boning.
Terms of use
Metadata
Show full item recordAbstract
In this thesis, we design and explore a new model architecture called a Variational Bayes Recurrent Neural Network (VBRNN) for modelling time series. The VBRNN contains explicit structure to disentangle time constant and time dependent dynamics for use with compatible time series, such as those that can be modelled by differential equations with time constant parameters and time dependent state. The model consists of a Variational Bayes (VB) layer to infer time constant state, as well as a conditioned-RNN to model time dependent dynamics. The VBRNN is explored through various synthetic datasets and problems, and compared to conventional methods on these datasets. This approach demonstrates effective disentanglement, motivating future work to explore the efficacy of this mo del in real word datasets.
Description
This electronic version was submitted by the student author. The certified thesis is available in the Institute Archives and Special Collections. Thesis: M. Eng., Massachusetts Institute of Technology, Department of Electrical Engineering and Computer Science, 2019 Cataloged from student-submitted PDF version of thesis. Includes bibliographical references (pages 85-86).
Date issued
2019Department
Massachusetts Institute of Technology. Department of Electrical Engineering and Computer SciencePublisher
Massachusetts Institute of Technology
Keywords
Electrical Engineering and Computer Science.