Longitudinal VoxelMorph : spatiotemporal modeling of medical images
Author(s)
Chambers, Adelaide Woods.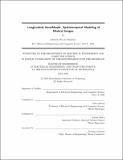
Download1192539460-MIT.pdf (1.941Mb)
Alternative title
Spatiotemporal modeling of medical images
Other Contributors
Massachusetts Institute of Technology. Department of Electrical Engineering and Computer Science.
Advisor
John Guttag and Adrian Dalca.
Terms of use
Metadata
Show full item recordAbstract
Medical image registration is an important initial step in many downstream clinical tasks. Repeated imaging for diagnostic, therapeutic, or scientific discovery is common. Classical longitudinal image registration systems are too slow to be useful in practice and are often only designed for a highly specific type of image data. Efficient pairwise image registration models are limited by not accounting for the temporal nature of the data. We present Longitudinal VoxelMorph, a novel machine-learning-based model for efficient and scalable spatiotemporal medical image registration. We also dene a new evaluation metric to quantify the temporal smoothness of a longitudinal deformation field. We evaluate the model on cardiac cine-MRI data and echocardiography data, and nd that Longitudinal VoxelMorph is more temporally consistent than state-of-the-art pairwise models, and achieves comparable or improved anatomical accuracy. Longitudinal VoxelMorph has the potential to be incorporated in downstream medical image tasks, such as image prediction and diagnosis, facilitating better clinical outcomes.
Description
Thesis: M. Eng., Massachusetts Institute of Technology, Department of Electrical Engineering and Computer Science, May, 2020 Cataloged from the official PDF of thesis. Includes bibliographical references (pages 67-76).
Date issued
2020Department
Massachusetts Institute of Technology. Department of Electrical Engineering and Computer SciencePublisher
Massachusetts Institute of Technology
Keywords
Electrical Engineering and Computer Science.