Relieving label requirements through weakly supervised learning
Author(s)
Chen, Bryan,M. Eng.Massachusetts Institute of Technology.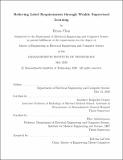
Download1192539594-MIT.pdf (3.613Mb)
Other Contributors
Massachusetts Institute of Technology. Department of Electrical Engineering and Computer Science.
Advisor
Jayashree Kalpathy-Cramer and Elfar Adalsteinsson.
Terms of use
Metadata
Show full item recordAbstract
Precise annotation of data is a time-consuming and costly process, yet many models rely on having large amounts of labeled data for training. Many domains, including healthcare, have access to large amounts of unlabeled data, motivating groups to leverage this data for training their machine learning models. This thesis firstly investigates the correlation between the size of a medical dataset and the performance of models for different machine learning tasks, such as classification, detection, and segmentation. This thesis further explores the utility of training with additional unlabeled data by adopting various weakly supervised techniques. Finally, while comparing these techniques to supervised learning baselines, we highlight the trade-offs between using smaller labeled data sizes and model performances.
Description
Thesis: M. Eng., Massachusetts Institute of Technology, Department of Electrical Engineering and Computer Science, May, 2020 Cataloged from the official PDF of thesis. Includes bibliographical references (pages 81-86).
Date issued
2020Department
Massachusetts Institute of Technology. Department of Electrical Engineering and Computer SciencePublisher
Massachusetts Institute of Technology
Keywords
Electrical Engineering and Computer Science.