Learning infection influence using self-excitatory temporal point processes
Author(s)
Kumar, Agni.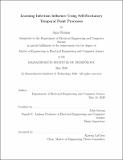
Download1192562368-MIT.pdf (1.507Mb)
Other Contributors
Massachusetts Institute of Technology. Department of Electrical Engineering and Computer Science.
Advisor
John Guttag.
Terms of use
Metadata
Show full item recordAbstract
Clostridioides difficile infection (CDI) is recognized as a leading cause of healthcare-associated infections in the United States. CDIs lead to poor health outcomes and impose a substantial burden on the healthcare system. Though hospitals across the country generally follow contact precautions for CDI, it has proved extremely difficult to control, as its transmission characteristics are not well understood. We propose using multi-task, multi-dimensional Hawkes processes (MMHPs), mathematical models with a self-exciting property, to learn CDI influence patterns over time. We discuss a robust optimization algorithm to learn MMHP models, in which we incorporate structural information directly into the objective function. Using data from a large urban hospital, we jointly model the dynamics of infection spread across multiple patient care units, systematically uncovering clustering structures among their individual influence patterns. Our experimental results demonstrate the efficacy of our approach and its utility in guiding unit-specific interventions aimed at curtailing the spread of CDI.
Description
Thesis: M. Eng., Massachusetts Institute of Technology, Department of Electrical Engineering and Computer Science, May, 2020 Cataloged from the official PDF of thesis. Includes bibliographical references (pages 63-67).
Date issued
2020Department
Massachusetts Institute of Technology. Department of Electrical Engineering and Computer SciencePublisher
Massachusetts Institute of Technology
Keywords
Electrical Engineering and Computer Science.