Online, low-latency decision making for Fetal Magnetic Resonance Imaging with machine learning
Author(s)
Xu, Junshen(Computer scientist)Massachusetts Institute of Technology.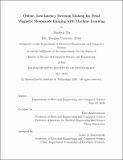
Download1192966407-MIT.pdf (4.539Mb)
Alternative title
Online, low-latency decision making for FMI with machine learning
Other Contributors
Massachusetts Institute of Technology. Department of Electrical Engineering and Computer Science.
Advisor
Elfar Adalsteinsson.
Terms of use
Metadata
Show full item recordAbstract
Fetal Magnetic Resonance Imaging (MRI) with T2-weighted Half-Fourier-Acquisition Single-Shot Turbo-Spin-Echo (HASTE) sequence plays an important role in diagnosing brain abnormality. However, the quality of HASTE images routinely suffer from fetal motion which leads to image artifacts, incomplete brain coverage as well as longer scan times. To address this problem, interleaved 3D Echo-planar Imaging (EPI) navigators are acquired along with HASTE images, which can provide pose information for prospective motion correction. In this thesis, we first propose a fetal pose estimation model which detects important fetal landmarks from 3D EPI data using a deep convolution neural network. We further demonstrate its capability by applying this model to fetal motion analysis. In an attempt to improve the current fetal MRI protocol, we develop a machine learning based online decision making system for fetal MRI to improve the efficiency of acquiring high quality HASTE images for clinical diagnosis. The proposed system leverages an Image Quality Assessment (IQA) network to determine whether an acquired HASTE slice is contaminated by motion artifacts and improves image quality by re-acquisition. Evaluation on retrospective experiments and in vivo scans suggests that the proposed pipeline can improve image quality with a reasonable number of re-acquisition, potentially enabling a more efficient workflow for fetal brain MRI.
Description
Thesis: S.M., Massachusetts Institute of Technology, Department of Electrical Engineering and Computer Science, May, 2020 Cataloged from the official PDF of thesis. Includes bibliographical references (pages 63-68).
Date issued
2020Department
Massachusetts Institute of Technology. Department of Electrical Engineering and Computer SciencePublisher
Massachusetts Institute of Technology
Keywords
Electrical Engineering and Computer Science.