On anomaly detection in particle accelerators
Author(s)
Sarda, Nilai Manish.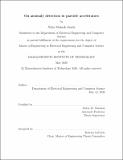
Download1193029286-MIT.pdf (7.992Mb)
Other Contributors
Massachusetts Institute of Technology. Department of Electrical Engineering and Computer Science.
Advisor
Justin M. Solomon.
Terms of use
Metadata
Show full item recordAbstract
In this thesis, we explore concepts related to anomaly detection at particle accelerators. In an extremely high-energy proton-proton collision, sprays of subatomic particles are generated and destroyed at extremely short timescales. Detecting anomalies in these event signatures is a crucial step in experimentally verifying new theories of physics beyond the Standard Model. Towards this end, we analyze the geometric structure of event signatures, which can be represented as discrete distributions of energy on the surface of a cylinder. Therefore, we approach the problem from the framework of optimal transportation. The optimal transport distance is the map between two measures which minimizes the total work required to transform one measure into another. First, we provide theoretical improvements in learning with transport-type distances. We show that minimizing the transport distance also leads to a coreset with respect to a broad class of functions, by showing a bound on the quadrature error of a Monte Carlo integration. In addition, we develop an unbiased estimator for a Gaussian kernel based on the sliced Wasserstein distance, which is based on the one-dimensional version of optimal transport. Next, we use these kernels within the framework of discriminative anomaly detection. The methods we consider apply transport distance-based kernels to classify anomalies on an event-by-event basis. We apply these techniques to two datasets, one from the field of particle physics and one inspired by biology. This comparison allows us to argue empirically which models are most effective for which types of anomalies. Finally, we move to the generative setting, and build a topic model based on the dijet factorization theorem to perform anomaly detection and quark/gluon discrimination. This approach leverages the fact that each jet in a dijet pair is statistically independent, and uses matrix factorization to disentangle the component distributions.
Description
Thesis: M. Eng., Massachusetts Institute of Technology, Department of Electrical Engineering and Computer Science, May, 2020 Cataloged from the official PDF of thesis. Includes bibliographical references (pages 123-136).
Date issued
2020Department
Massachusetts Institute of Technology. Department of Electrical Engineering and Computer SciencePublisher
Massachusetts Institute of Technology
Keywords
Electrical Engineering and Computer Science.