Towards a cognitive network management and control system
Author(s)
Rezaee, Arman.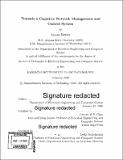
Download1201526158-MIT.pdf (16.52Mb)
Other Contributors
Massachusetts Institute of Technology. Department of Electrical Engineering and Computer Science.
Advisor
Vincent W.S. Chan.
Terms of use
Metadata
Show full item recordAbstract
Future networks have to accommodate an increase of 3-4 orders of magnitude in data rates with heterogeneous session sizes and strict time deadline requirements. The dynamic nature of scheduling of large transactions and the need for rapid actions by the network management and control system, require timely and judicious collection of network state information. Within this context we will focus on the problem of shortest path routing, and identify pragmatic schemes that allow a central controller to collect relevant delay statistics from various links and nodes within the network. We present Significant Sampling as an adaptive monitoring technique to collect and disseminate network state information when it can be of significant value to the optimal operation of the network, and in particular when it can help in identifying the shortest routes. We start by developing an analytical framework that can identify the optimal time for the collection of such information in a small but realistic setting, when the underlying delay model is a continuous-time diffusion process (e.g. Wiener process or Ornstein-Uhlenbeck process) and its parameters are known by the controller. We show that this technique balances the need for updated state information against the collection and dissemination costs and provides an algorithm that yields near optimum performance. We then extend the results by introducing a reinforcement learning framework that learns the aforementioned optimal policy from its own interactions with the network, and without any prior assumptions regarding the underlying delay model. In addition to achieving a performance comparable to the analytically derived policies, the deep reinforcement learning solution is more flexible and general and can accommodate a diverse set of network environments. This is particularly important because it can provide good solutions for complex network environments where analytically tractable solutions are not feasible. We conclude our work by noting that sensible network controllers should continue to deliver a good performance between distinct instances of state collection and thus any meaningful solution should strive to meet application demands despite the unavoidable uncertainty about the instantaneous state of the network. To that end, we introduce a novel diversity routing scheme that can accommodate requirements regarding delay variations despite a controller's relative uncertainty about the instantaneous state of the network. More specifically we utilize mean-variance analysis as the basis for traffic distribution and route selection, and show that this technique can improve the users' quality of service by taking into account the correlated nature of delay across different paths. We conclude this work by commenting on the potential application of this method to general transportation networks.
Description
Thesis: Ph. D., Massachusetts Institute of Technology, Department of Electrical Engineering and Computer Science, 2020 Cataloged from PDF of thesis. Includes bibliographical references (pages 155-159).
Date issued
2020Department
Massachusetts Institute of Technology. Department of Electrical Engineering and Computer SciencePublisher
Massachusetts Institute of Technology
Keywords
Electrical Engineering and Computer Science.