Synthesizing tabular data using conditional GAN
Author(s)
Xu, Lei(Electrical and computer scienctist)Massachusetts Institute of Technology.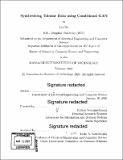
Download1202001437-MIT.pdf (7.589Mb)
Other Contributors
Massachusetts Institute of Technology. Department of Electrical Engineering and Computer Science.
Advisor
Kalyan Veeramachaneni.
Terms of use
Metadata
Show full item recordAbstract
In data science, the ability to model the distribution of rows in tabular data and generate realistic synthetic data enables various important applications including data compression, data disclosure, and privacy-preserving machine learning. However, because tabular data usually contains a mix of discrete and continuous columns, building such a model is a non-trivial task. Continuous columns may have multiple modes, while discrete columns are sometimes imbalanced, making modeling difficult. To address this problem, I took two major steps. (1) I designed SDGym, a thorough benchmark, to compare existing models, identify different properties of tabular data and analyze how these properties challenge different models. Our experimental results show that statistical models, such as Bayesian networks, that are constrained to a fixed family of available distributions cannot model tabular data effectively, especially when both continuous and discrete columns are included. Recently proposed deep generative models are capable of modeling more sophisticated distributions, but cannot outperform Bayesian network models in practice, because the network structure and learning procedure are not optimized for tabular data which may contain non-Gaussian continuous columns and imbalanced discrete columns. (2) To address these problems, I designed CTGAN, which uses a conditional generative adversarial network to address the challenges in modeling tabular data. Because CTGAN uses reversible data transformations and is trained by re-sampling the data, it can address common challenges in synthetic data generation. I evaluated CTGAN on the benchmark and showed that it consistently and significantly outperforms existing statistical and deep learning models.
Description
Thesis: S.M., Massachusetts Institute of Technology, Department of Electrical Engineering and Computer Science, February, 2020 Cataloged from PDF version of thesis. Includes bibliographical references (pages 89-93).
Date issued
2020Department
Massachusetts Institute of Technology. Department of Electrical Engineering and Computer SciencePublisher
Massachusetts Institute of Technology
Keywords
Electrical Engineering and Computer Science.